A Brief History of Diffusion AI-Heart of Modern Image Generation
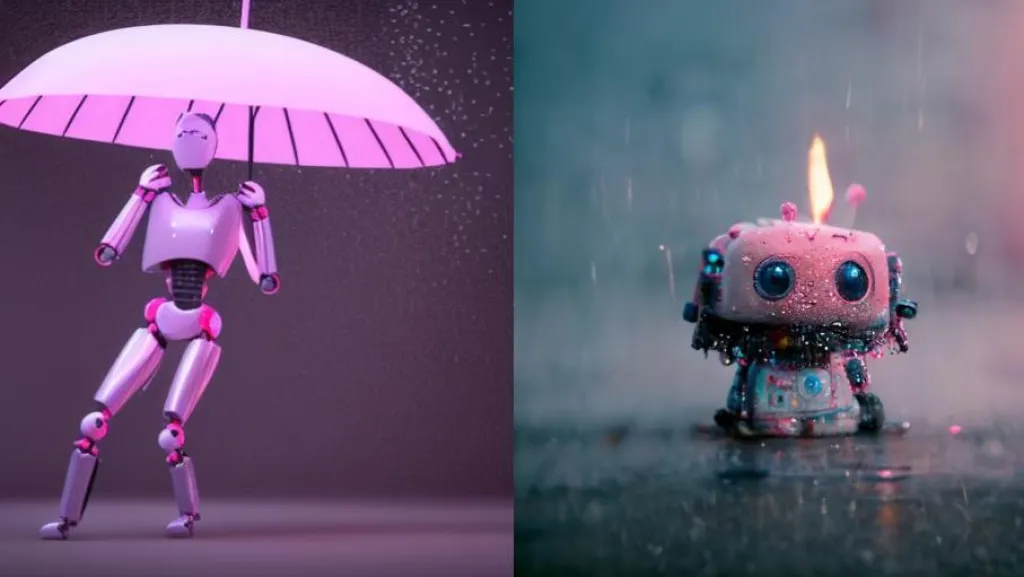
Text-to-image As technological advancements substantially improved the fidelity of art that AI systems could generate, AI surged this year. Although controversial, methods like Stable Diffusion and OpenAI’s DALL-E 2 have been embraced by platforms like DeviantArt and Canva to power creative tools, customize branding, and even come up with new product ideas. But these systems’ core technology is capable of much more than just producing art. It is known as diffusion, and some daring research teams are using it to create music, construct DNA sequences, and even find new medications. Let’s take a look at the brief history of Diffusion AI!
The Birth of Diffusion AI
You may remember the deepfaking app craze that was popular a few years ago. These applications used people’s portraits to replace the original subjects in target content in existing images and movies. The apps would “insert” a person’s face, or in some cases, their entire body, into a scene using artificial intelligence, frequently convincingly enough to fool a person at first glance.
Generative adversarial networks, or GANs for short, is an AI technology that was used by the majority of these applications. GANs are made up of two components: a generator that creates artificial examples (such as photographs) from random data and a discriminator that tries to tell the difference between the artificial instances and actual examples from a training dataset.
Both the generator and discriminator get better at what they do. This is until the discriminator can no longer distinguish between genuine cases and synthesized ones with more than the 50% accuracy anticipated from chance.
Top-performing GANs can produce images of made-up residential structures, for instance. In order to create high-resolution head images of fictional persons, Nvidia built a system called StyleGAN that can learn characteristics such as facial position, freckles, and hair. GANs have been used to create vector sketches and 3D models in addition to images. This demonstrates their ability to produce speech, video clips, and even song instrument loops.
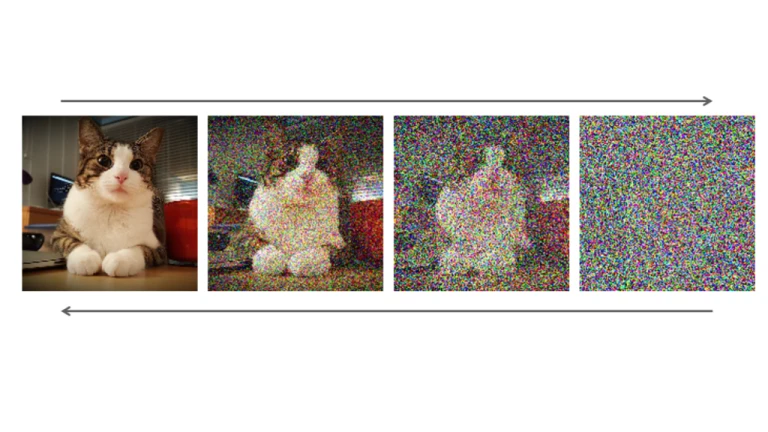
However, because of their design, GANs had a number of drawbacks in actual use. The training of generator and discriminator models simultaneously was intrinsically unstable; on occasion, the generator “collapsed” and output large numbers of samples that appeared to be similar. GANs were difficult to scale since they required a lot of data and computational resources to run and train.
Enter Diffusion AI.
How Diffusion AI Works
A sugar cube dissolving in coffee is an example of how something can travel from a region of higher concentration to one of lower concentration. This is how diffusion was influenced by physics. Coffee contains sugar granules that are first concentrated at the top of the beverage before dispersing over time.
Diffusion systems notably take inspiration from diffusion in non-equilibrium thermodynamics. This is where the process over time raises the entropy, or randomness, of the system. Consider a gas: through random motion, it will eventually diffuse to uniformly fill a room. Similar to how data like photos can become a uniform distribution by introducing noise at random.
Diffusion systems gradually erode the structure of data by introducing noise until there is just noise remaining.
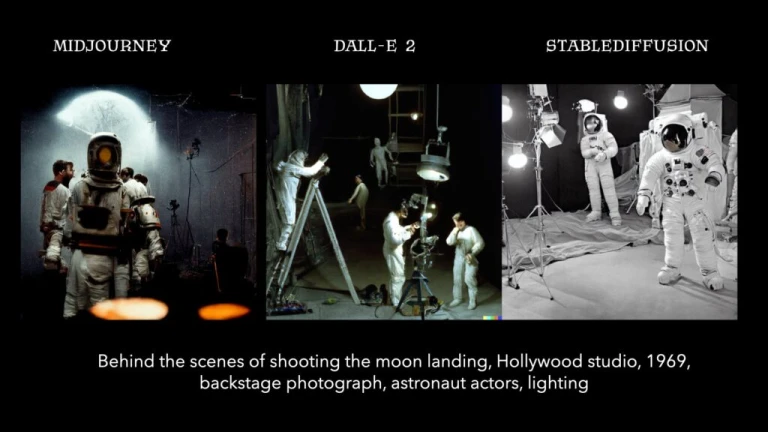
Systems for diffusion have been in use for almost ten years. However, they have become much more useful in real-world applications thanks to a relatively recent breakthrough from OpenAI called CLIP. This is short for “Contrastive Language-Image Pre-Training”. In order to “score” each stage of the diffusion process depending on how probable it is to be classified under a specific text prompt, CLIP categorizes data, such as photographs.
The data initially has a very low CLIP score because it is primarily noise. But when the diffusion system extracts information from the noise, it gradually approaches the prompt. Uncarved marble makes a good comparison since CLIP directs the diffusion system toward images that will receive higher scores, just like a master sculptor would direct a novice.
Along with the DALL-E image-generating technology, OpenAI debuted CLIP. Since then, it has been incorporated into both DALL-E 2 and open source substitutes like Stable Diffusion.
What can it do?
What are the applications of CLIP-guided diffusion models? As was previously mentioned, they’re pretty adept in producing art, including photorealistic works as well as sketches, drawings, and paintings in virtually any style. In fact, research suggests that they repeat part of their training data in an unsatisfactory manner.
Although it may be debatable, the models’ talent doesn’t stop there.
Additionally, scientists have tried composing new music using directed diffusion models. An organization called Harmonai, which receives funding from Stability AI, the London-based startup that created Stable Diffusion, has unveiled a diffusion-based model. This can produce music clips after being trained on hundreds of hours of previously published songs.
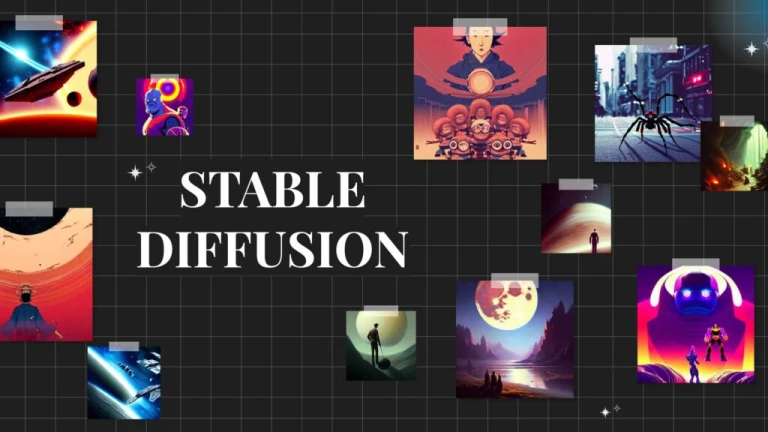
More recently, programmers Seth Forsgren and Hayk Martiros came up with the hobby project Riffusion, which cleverly trains a diffusion model using spectrograms, which are visual representations of music, to produce tunes.
Diffusion technology is being applied to biomedicine outside of the music industry. This has been done by a number of labs in an effort to find novel disease treatments. As MIT Tech Review reported earlier this month, startup Generate Biomedicines and a team from the University of Washington developed diffusion-based models to generate designs for proteins with particular characteristics and functionalities.
The models operate in various ways. By disassembling the chains of amino acids that make up proteins, Generate Biomedicines introduces noise. Random chains are then combined to create a new protein under the direction of the researchers’ defined limitations.
The Future
What might the diffusion model’s future hold? The possibilities are likely endless. It has already been used by researchers to create films, compress photos, and synthesize speech. That doesn’t mean that, like GANs with diffusion, a machine learning technique that is more effective and performant won’t eventually replace diffusion. But there’s a reason it’s the fad architecture right now; dissemination is nothing if not adaptable.
Follow us on Instagram: @niftyzone